uncategorized
AI Infancy and Evolution
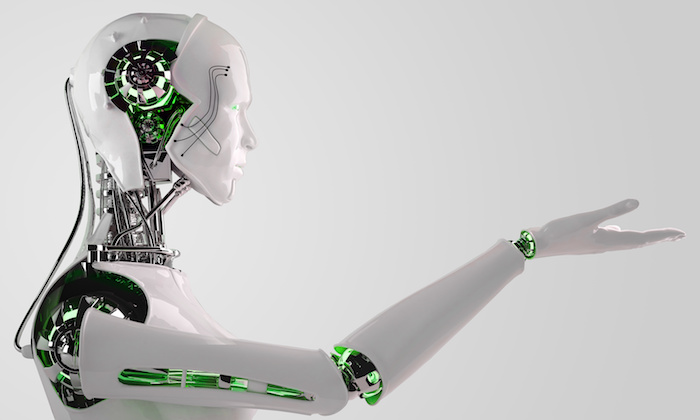
As the world continues to get more complex in every facet, we are starting to see AI become assistive to help people in their roles. Utility companies can use drone imagery and thermal mapping in conjunction with AI data to detect and anticipate equipment failure. IBM Watson is helping doctors condense mass amounts of data into something digestible. Many companies are beginning to take advantage of the benefits AI systems can offer, but one thing remains the same, we are only scratching the surface.
For sales teams, there have been countless enablement tools that make big claims. Traditional AI tools typically use historical data to generate a “predictive” information analysis to help you shape future results. While that is a good first step, it falls short in a few ways.
Not enough data to make a good assessment.
Data entry is not a highly motivating task. While Accent Technologies allows for automated data entry, there may not be enough data in your CRM to make a good correlation to predict results. That is not any one individual’s fault, it is a collective book of reasons why there may not be enough data. Nonetheless, the lack of data may be prohibitive in moving forward with today’s infant AI/predictive tools.
The world keeps changing.
Which means there might be enough data, but sales strategies are always changing. What is considered to be good business today may not be good business going forward. Maybe the organization has paid for studies that are telling it to go in a different direction or focus in a certain industry or market. Or maybe you just need to make adjustment so your sales team can focus on more attractive business, low hanging fruit, more profitable business or beyond that. The point is that strategies change, and models have to allow an organization to create success first. Then machine learning can help amplify those successes throughout the organization.
Not having quality data.
For example, and as an exaggeration of the example: if you had 100 sales people and they were all “A” players and they all forecasted deals 2 weeks before they were considered a “win” and subsequent closure of the deal, you may have bad quality data to be predicting future events on. This is, of course, an exaggerated example but it can go many different ways. Sales reps may be putting in more than what is real, potentially “forecasting” deals that may not be actually progressing. Additionally, reps might be successful in areas that may no longer be strategically valuable to the company. Whatever the case is, there needs to be a check for the quality of data.
The models are based only on historical quantitative big data (or just data).
What about the “thick data”? The human insight that can’t be quantified. Tricia Wang, a Technology ethnographer, discusses this at length and why it is important to combine big data with thick data so you can provide the lost context surrounding the quantitative findings. This goes back to the point made in number 2. We have to allow adjustment of the models through machine learning or manual intervention.
The bottom line is predicting what might close and prioritizing opportunities are two separate endeavors. Probability should be taken into consideration, but other variables (both quantitative and qualitative) should also be considered. These variables can range from a number of different categories like sales productivity, sales performance management, compensation planning tools, optimization tools, coachability of the sellers, etc.
Accent Technologies has determined that long-term success requires short-term adjustments. We design our standard models with the capability to be flexible out of the box. This allows teams to make adjustments as they go through deployment or make strategic changes in the future. With a flexible algorithm that can be easily adjusted, your human experts can inject that “thick” data into the equation which will ultimately bring you the most value . We also leverage different data sources to ensure there is sufficient quantitative data flowing. Understanding your historical data, where you want to go and how to get there, will lead the way in effective use of AI. Giving the steering wheel to the organization is a part of what we do and how we help companies execute their strategy.