uncategorised
What is Prescriptive Analytics?
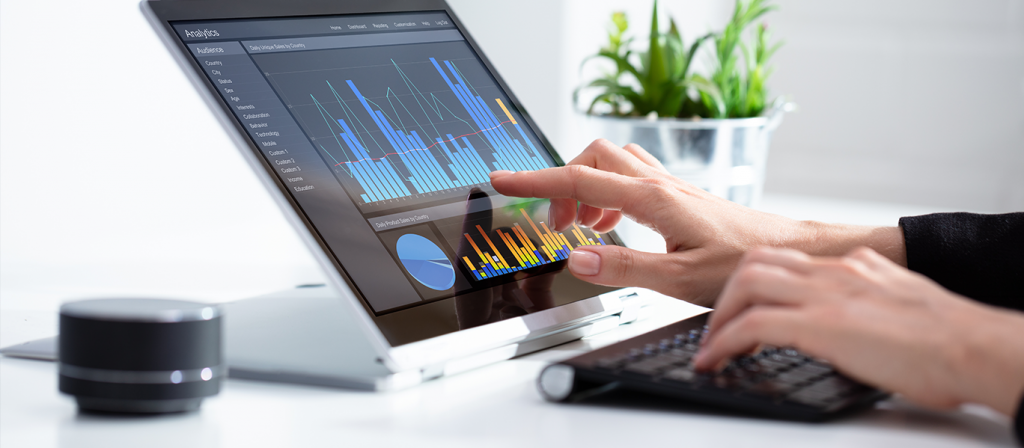
How to use technology to predict and respond to the behavior of your future customers.
Welcome to the wide world of business analytics. Business analytics is using data to inform business decisions. It is also sometimes referred to as business intelligence.
The scope of business analytics has grown significantly. Especially as technology and artificial intelligence (AI) have become more powerful and accessible.
There’s a reason data scientists are in high demand. They are professionals trained to wrangle, visualize, and interpret large amounts of data.
There are full-time positions (sometimes even whole departments) dedicated to data science. They analyze customer behavior, browse patterns, and buying sentiment. Almost everything can be tracked, analyzed, and acted upon to gain a competitive edge. From broad buying decisions to granular website behavior.
Before we dive in and figure out what analytics are all about, let’s take a brief look at three other modes of analysis. These serve as the fundamental building blocks of prescriptive analytics.
Types of business analytics
The scope of this post will stay within the bounds of prescriptive analytics. But if you want a deep dive into all four of the main types of business intelligence, be sure to check out some of our other posts on the topic.
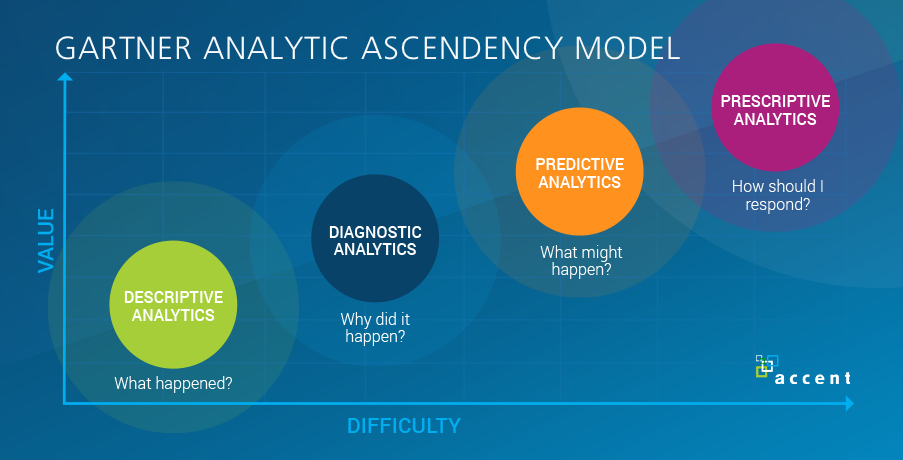
SEE ALSO: What is Business Analytics?
SEE ALSO: Examples of Prescriptive Analytics
SEE ALSO: Stop Confusing Predictive and Prescriptive Analytics.
In summary, here are the four main types of business analysis:
- Descriptive analytics (cleaning and visualizing data of past events)
- Diagnostic analytics (teasing out correlations and creating hypotheses based on past events)
- Predictive analytics (using conclusions from past events to try to predict future events)
- Prescriptive analytics (prescribing or executing the best possible action based on the predicted future)
The first two types of analysis (descriptive and diagnostic) are reactive analytics. Nothing in the future changes, and the data is strictly rear-facing. They deal with the past.
It’s important to understand reactive analytics are not inferior to proactive analytics. They’re foundational and necessary to build upon. They don’t provide answers for actions to take in the future. Yet without them predictive and prescriptive analytics could not exist.
What is prescriptive analytics?
Prescriptive analysis involves running predictive data through complex simulation algorithms. Then outputting the best result for the end user. Daniel Bachar from LOGILITY summarizes it well:
“At their best, prescriptive analytics predict not only what will happen, but also why it will happen, providing recommendations regarding actions that will take advantage of the predictions.”
So, the answer to the question “what are prescriptive analytics?” is the highest and most coveted form of business analytics. It is the ultimate goal of all prior analysis.
Not only does this form of analysis study the past and predict the future, but as the name implies, “prescribes” the most appropriate actions to take.
On its face, it seems like a superpower. All we need to do is input data and some sort of machine spits out exactly what action we need to take to be successful? If it’s that easy, why isn’t everyone doing it?
There are challenges to implementing this kind of analysis. It’s one of the most up-and-coming ways businesses are edging out the competition.
Gartner anticipates the prescriptive analytics software market will continue to grow.
They predict it will experience a 20.6 PERCENT CAGR between 2017 and 2022. This suggests that almost 37 percent of businesses will start using prescriptive analytics.
Certain organizations are even beginning to process unstructured data. Examples of unstructured data include photos, videos, or music files. They do this to inform and predict user behavior. Google Photos, for example, has facial recognition software. It will detect the people present in your uploaded photos and sort them into specific albums.
As you continue to sort faces into albums, the app “learns” how to best analyze the faces of your friends and family. This information makes future sorting easier.
But how does it all work? And how can it help your organization best respond to the future?
How does prescriptive analytics work?
There are many ways to use prescriptive data analytics. But all technologies and methods boil down to three crucial mechanisms:
- A mechanism to store and visualize past data
- A mechanism to use that data to predict the future
- A mechanism to interpret this predicted future and best respond to it
This is a simple summary. A lot of technology, manpower, and effort go into executing these three steps.
It’s clear why computers and AI are the tools of choice for prescriptive analytics. To compute all the data and produce accurate results by hand is unfathomable. It’s an impossible task no matter how skilled your team is.
Another relevant example of prescriptive analytics in action is WAYMO. Waymo is Google’s self-driving car. Even the tagline of their website is “We’re building the World’s Most Experienced Driver.” This language is no accident.
The incredible thing about AI is that it’s always learning, growing, and — as Google says — “building”. That is true both in the world of self-driving cars and sales and marketing. It never stops, much how humans never stop learning as they grow older.
As you dive deeper into prescriptive analytics, you may hear the term “neural network.” This refers to the way a machine learns, which mirrors how a child learns. Every new piece of data creates a virtual connection in the “brain” of the machine. This increases the machine’s ability to interpret new data.
This “machine learning” technology isn’t a specific coding language or application. It takes many different forms, from self-driving cars to sales enablement platforms.
It helps determine when a car should slow down, brake, or speed up. It can tell you when a customer is most primed for an inside sales rep to call. Every piece of data fed into modern AI models increases the probability of success in the output.
These suggestions or prescribed solutions are based on ever-evolving predictive models and historical data. The data is always compared against historical behavior. This practice produces the best possible result.
It’s like something out of a science fiction novel. But it’s also great for business.
The Pros and Cons of Prescriptive Analytics
Like many things in life, there are both pros and cons when it comes to using prescriptive analytics in your business. Let’s break down some of the most common benefits and issues before we dive into how these tools can transform your business.
Pro: Make informed, data-driven decisions
One of the key areas where prescriptive analytics shines is in the decision-making process. In the old days, company’s often made decisions based on instinct, intuition, and gut feelings.
Sometimes that worked to their advantage. Sometimes it didn’t.
Prescriptive analytics removes a lot of the emotional guesswork out of the decision-making process by giving you real world data. Analyzing and understanding that data will allow you to make better decisions.
Would you rather guess what the next year holds for your business or would you prefer to make that decision based on actual metrics gathered and analyzed by artificial intelligence? Prescriptive analytics will help you do the latter.
Pro: Simulate probability to reduce risk
Building on the previous point, you’ll be able to make better-informed decisions because prescriptive analytics examines huge amounts of data and then determines the probability of outcomes based on that information.
Understand the likelihood of success (or failure) before you make a decision will help you avoid pitfalls and lost revenue, while also helping you spot opportunities you might have missed.
Pro: Increase efficiency
Finally, by understanding probability and using prescriptive analytics to make informed decisions you’ll be better equipped to make decisions faster. This will lead to increased efficiency.
Think about it. How many times has your business faced a big decision? During those times, you probably spent days (or weeks…or months) having meetings, doing research, having discussions, and losing sleep at night trying to decide what to do.
All that time is time you could spend doing other things.
And with prescriptive analytics, you can get that time back. You’ll always have detailed data and analytics at hand when it’s time to make a decision. With this clearer understanding of the big picture, you’ll make decisions faster and can get back to focusing on other things.
Con: Only effective with valid input
Prescriptive analytics tools can break down huge amounts of data and help you make informed decisions. The catch is that the recommendations are only as good as the data you feed the machine.
To be truly effective, you’ll need to be on top of your data sources. Vet them. Re-evaluate regularly. Remove outliers.
You need the best data to get the best results.
Con: Not as reliable for long-term decisions
Prescriptive analytics can feel a bit like having your own personal crystal ball, there are limitations.
The tools and predicted outcomes are most accurate and reliable in the short-term. As your timeline grows, so does unpredictability.
This isn’t the fault of prescriptive analytics as much as it is just a simple fact of life: it’s harder to predict things far in the future because the amount of variables involved can increase.
Con: Not all prescriptive analytics providers are legit
As big data has grown, so too has the number of companies offering services in this space.
On the plus side, more choice and options is always better. It keeps prices lower and increased competition leads to faster technological advancements.
On the other hand, there are a lot of companies in this space who cash in on the excitement of big data without providing any real service.
Because of this, it’s imperative you vet your analytics providers. Schedule demos. Ask questions. Do your research. Talk with other customers if possible.
Signing a software contract is a big commitment. Make sure you’re finding the right fit for your company.
Additional Benefits of Prescriptive Analytics
We’re often asked “why are prescriptive analytics important?”, so let’s highlight a few more of the lesser known benefits of prescriptive analytics.
Map a path to success
Finding the way forward in the modern business world can be a challenge. With prescriptive analytics, you can remove the guesswork and develop a roadmap to success.
Because these tools take a lot of the guesswork out of your business, you’ll be able to formulate plans based on real probability – not hunches and wishful thinking.
Inform real-time and long-term business operations
With the insights provided by prescriptive analytics, you’ll be able to improve your business operations across the board. Both today and in the long-term.
This is possible because prescriptive analytics will allow you see your business both at the macro and micro levels. Armed with this information, it’s easier to spot areas for improvement.
Spend less time thinking
We touched on this one earlier, but with prescriptive analytics you’ll get data-backed insights into your operation. This means you’ll have to spend less time playing “what if?” with every major decision.
Freed from this, you’ll be able to spend more time implementing things that can improve your business.
Reduce human error and bias
Finally, by using prescriptive analytics tools, you’ll greatly reduce human error and bias in the decision-making process.
The AI in these tools looks at data and makes decisions based on the information it has compiled. It doesn’t have an agenda. It’s not swayed by emotion. It makes decisions based on science.
When the AI recommends something, you can be sure it’s based on data.
Common prescriptive analytics technologies
We’ve already touched on neural networks. Other common technologies used for prescriptive analytics include the following:
- Linear, time-based, and logistic regression simulations
- Complex event processing
- Recommendation engines
- Heuristics
- Machine learning
- Naïve Bayes conditional probability
If your main focus is sales, it’s rare that you’ll run across any of these “in the wild.” These are often back-end technologies baked into software solutions or platforms.
For example, our sales content management platform ACCENT CONNECT offers intelligent content suggestions based on individual sales situations. Our technology utilizes machine learning to sift through situational variables. It also locates the most relevant content for the prospect.
Examples of prescriptive analytics
Here are a few industry-specific examples of how prescriptive analytics impact today’s marketplace. To find an even more detailed article on this topic, check out this article.
Prescriptive analytics in banking
Ever received a text from your bank notifying you of potential fraudulent charges? This process isn’t usually performed by humans. It’s monitored by complex AI technologies. These AIs study, predict, and prescribe solutions based on prior charges.
Companies like American Express and CitiBank use sophisticated predictive models. They use them to forecast and prevent customer churn. Machine learning can understand when cardholders are likely to switch to a different bank. Thus, they can respond with discounts, promotional credit, and other incentives.
Prescriptive analytics in retail
There’s one form of prescriptive analytics so commonplace, you’ve likely seen it without even realizing it. It is, of course, Amazon’s recommendation engine. Everything you see on Amazon’s homepage comes from your buying and browsing patterns.
Carousels with titles like “Inspired by your Wish List” and “Books You Might be Interested In.” And 99% of the time, they’re spot on. How can this be?
Amazon analyzes your data and cross-references your purchasing patterns against other like-minded buyers. It does this to create up-sell and cross-sell opportunities. This system is so intelligent, it can even transcend whole categories.
If you buy a book on landscape photography, it won’t only offer up another book on photography. It will offer up a new camera travel bag and wide-angle lens.
When it comes to retail, many businesses are replicating what Amazon has pioneered. Still, Jeff Bezos’ creation still remains the gold standard for how to use data to drive business.
Prescriptive analytics in healthcare
The prescriptive model of decision making is often used by hospitals and large healthcare networks. They’re used to improve patient outcomes. Historical data and predictive models are analyzed to determine the cost-effectiveness of certain medical procedures. They can even determine which patients have the highest risk of readmission or relapse.
Everything from medication prices to the efficacy of high-risk surgeries gets analyzed. You can also use them to create better solutions for patients and healthcare organizations.
Prescriptive analytics in education
Public schools and universities have a more challenging time utilizing prescriptive analysis. But the online education space is way ahead of the curve. Online education marketing is projected to reach $350 billion by 2025 globally. This is no surprise. The
inexpensive, scalable model of sites like Skillshare, Lynda.com, Khan Academy, Udemy, Teachable, and countless others are beginning to make traditional college degrees look obsolete.
These sites study user behavior to find gaps in their skillset. Then it creates programs to close those gaps to help them succeed in the workforce. For example, imagine if a user shows interest in web development or JavaScript. In this case, the AI may recommend a course on data science next.
If the user shows no interest in the recommendation, the platform may recommend a course on app development. All this serves to help the AI learn interest patterns and common career trajectories for future users.
Sites like this can also reduce churn by detecting when users are inactive and recommending a new and exciting course to keep them engaged.
Prescriptive analytics in travel
Airline companies are often masters of prescriptive analytics. This is why there is such a dramatic variance in the cost of air travel. AI systems are always analyzing variables such as weather, customer demand, time of year, even gasoline prices. The resulting data affects ticket prices on a day-by-day basis.
It’s more complex than ticket prices being high during the holidays and low during the working months. There is an entire AI-powered economy happening under the hood of most airline organizations.
Key takeaways of prescriptive analytics technology
Before we wrap things up, let’s take a few moments to highlight the key takeaway of prescriptive analytics technology.
If you need to pitch the features and benefits of this technology to your C-level managers, these are the points to focus on:
- Increases worker efficiency
- Provides a clear roadmap to growth and increased profitability
- Removes guesswork and emotion from the decision-making process
- Provides more accurate forecasting
- Highlights growth opportunities you may have missed
- Presents macro and granular views of your business
- Technology pays for itself with increased profitability
How you can use prescriptive analytics technology
When it comes to sales enablement and marketing, prescriptive analytics is your best friend.
Data is the lifeblood of customer behavior. The most successful sales teams understand this. They embrace prescriptive analysis as a powerful competitive advantage.
Imagine being able to articulate prospects’ pain points better than they can. Imagine knowing exactly when to place a phone call to a potential buyer at the precise time that they’re the most likely to buy.
However, the further up the hierarchy you go (from descriptive, to diagnostic, to predictive, to prescriptive), the more challenging the analysis becomes to execute manually. Without technology and software, scaling your business intelligence beyond one customer is a fool’s errand.
That’s why we’ve built AI-DRIVEN SALES ENABLEMENT TOOLS that automate the hard work of business analytics. Additionally, they provide prescriptive solutions that help you sell more efficiently.
The software does the hard work of predictive models, algorithms, and computations. That way, your team can get back to selling.
To learn more about how you can utilize prescriptive analytics to understand your future customers better and close more deals, SIGN UP FOR A FREE DEMO OF OUR PLATFORM.
By Accent Technologies
29th May 2020
Prescriptive Analytics the Accent Way
Sales data analysis is not easy for anybody—even experienced managers. But AI is great at harvesting relevant information from your data sources and conducting systematic pattern analyses to produce insights.
Leveraging AI in your sales practice will ensure you gather and visualize the data you need to effectively score your buyer engagement, your seller performance, and your pipeline’s movement forward.
It can also protect your sales forecasts from the inaccuracies that manual gathering (AKA subjective interpretations) presents. It elevates your strategy grounding it in data, rather than a “feeling.” Contact us today to see Accent’s Sales AI in action.